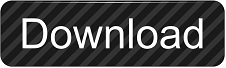

DeepAb predicts a set of inter-residue geometric constraints that are fed to Rosetta to produce a complete F V structure 14. Meanwhile, antibody-specific deep learning methods such as DeepAb 14 and ABlooper 15 have significantly improved CDR loop modeling accuracy, including for the challenging CDR H3 loop 7, 16. However, performance on antibody structures remains to be extensively validated. Beyond monomeric proteins, AlphaFold-Multimer has demonstrated an impressive ability to model protein complexes 13.

With the development of AlphaFold, accurate protein structure prediction has largely become accessible to all 12. Given its central role in binding, advances in prediction of H3 loop structures are critical for understanding antibody-antigen interactions and enabling rational design of antibodies.ĭeep learning methods have brought about a revolution in protein structure prediction 10, 11. Further, the position of the H3 loop at the interface between the heavy and light chains makes its conformation dependent on the inter-chain orientation 8, 9. However, the third CDR loop of the heavy chain (CDR H3) has proven a challenge to model due to its increased diversity, both in sequence and length 6, 7. Five of the CDR loops tend to adopt canonical folds that can be predicted effectively by sequence similarity 5. Accurate modeling of these CDR loops provides insights into these binding mechanisms and promises to enable rational design of specific antibodies 4. The interactions that facilitate antigen binding are determined by the structure of a set of six loops that make up a complementarity determining region (CDR). However, sequence data alone provides only a partial view into the immune repertoire. Analysis of these repertoires can further our understanding of the adaptive immune response 2 and even suggest potential therapeutics 3. Immune repertoire sequencing provides a glimpse into an individual’s antibody population 1. Through genetic recombination and hyper-mutation, the adaptive immune system is capable of generating a vast number of potential antibodies. As a demonstration of IgFold’s capabilities, we predicted structures for 1.4 million paired antibody sequences, providing structural insights to 500-fold more antibodies than have experimentally determined structures.Īntibodies play a critical role in the immune response against foreign pathogens.
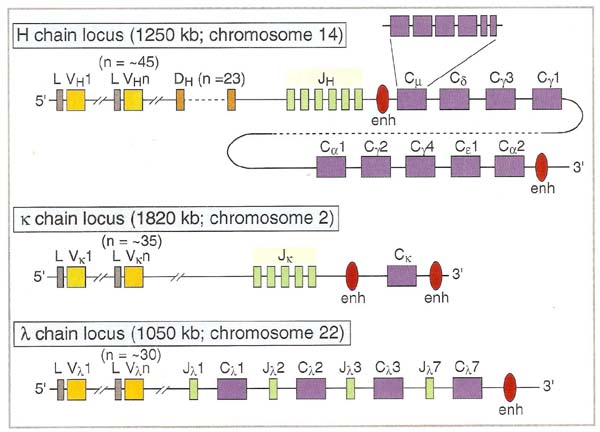
Accurate structure prediction on this timescale makes possible avenues of investigation that were previously infeasible. IgFold predicts structures of similar or better quality than alternative methods (including AlphaFold) in significantly less time (under 25 s). IgFold consists of a pre-trained language model trained on 558 million natural antibody sequences followed by graph networks that directly predict backbone atom coordinates. Here, we present IgFold, a fast deep learning method for antibody structure prediction. Even with recent advances, accurate structural prediction of these loops remains a challenge. The binding of antibodies is facilitated by a set of six hypervariable loops that are diversified through genetic recombination and mutation. Antibodies have the capacity to bind a diverse set of antigens, and they have become critical therapeutics and diagnostic molecules.
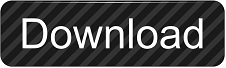